Moire Pattern Removal via Attentive Fractal Network (CVPR Workshop 2020) top 2 in Track2 NTIRE 2020
- Authors: Dejia Xu, Yihao Chu, Qingyan Sun
-본 논문에서는 영상의 Moire artifact를 제거하기 위해 AFN(Attentive Fractal Network)을 제안한다. AFN은 Encoder, AFB(Attentive Fractal Block), Decoder, Global Residual Learning 총 4가지로 구성된다. 이를 도식화하면 아래 equation 1과 같다.
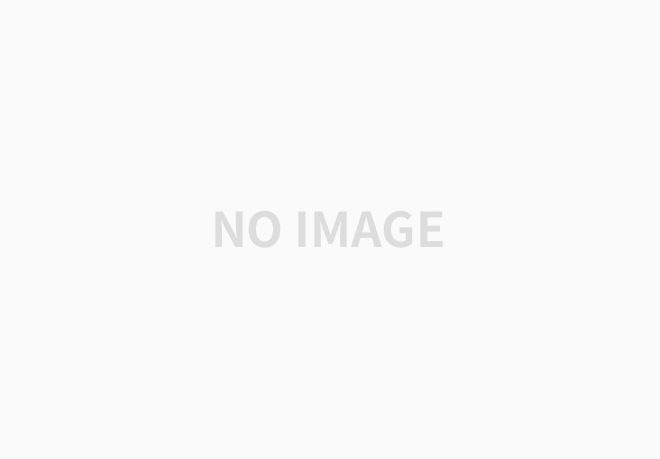
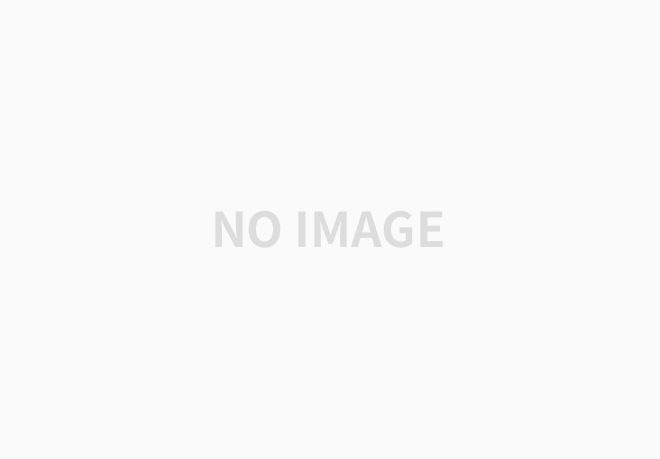
-모아레 이미지는 Encoder를 통해 multi-channel feature map이 되며 AFB에 입력된다. Encoded feature는 AFB를 통해 refinement되며 다시 Decoder를 통해 3-channel image가 되고 input image를 다시 합하는 global residual learning을 통해 네트워크를 안정화한다.
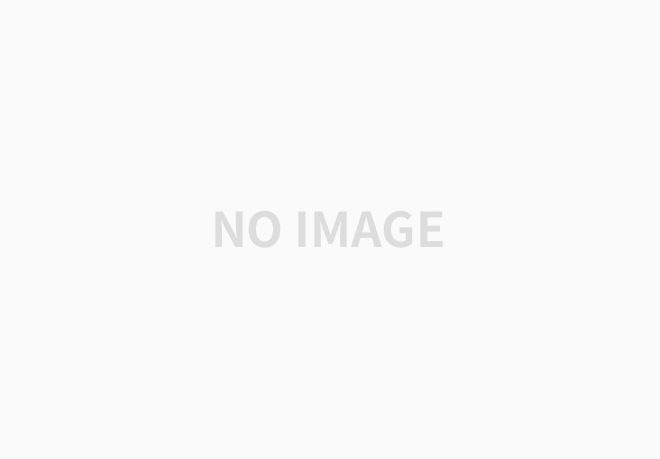
-High-level AFB는 각각 아래 Figure 3의 Low-level AFB로 구성된다. High-level AFB는 progressively feature fusion과 Channel Wise Attention 그리고 local residual learning으로 구성된다.
1) Progressively feature fusion는 점진적으로 초기 단계의 intermediate feature들을 전달하고 제일 마지막에 모두 fuse한다. 동일한 level의 feature들을 fuse함으로 서로 다른 stage들의 풍부한 information들이 network의 학습에 도움을 준다.
2) Channel Wise Attention은 과도한 양의 channel들이 network에 혼동을 줄 수 있기 때문에 SE(Squeeze and Excitation) block으로 adopt channel-wise attention을 진행한다. Learnable weight를 곱하며 fusion feature map의 weight는 essential한 feature들에 초점을 두며 re-weight가 진행된다.
3) Local Residual Learning은 network의 안정화를 위해 사용된다.
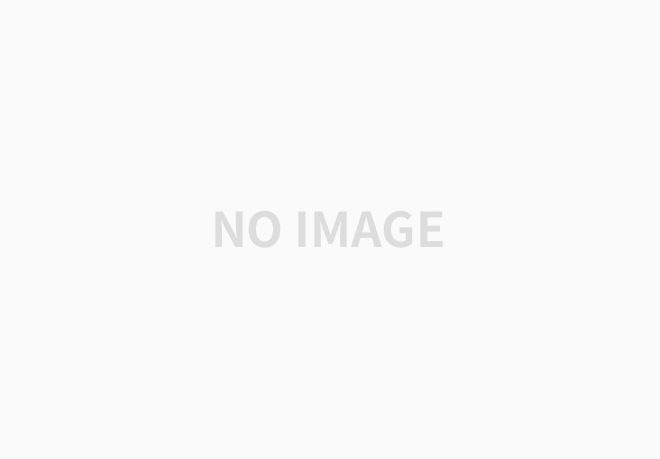
-Low-level AFB는 convolution layer와 Leaky ReLU를 지난다. 더불어 Local Shortcut를 통해 network의 부담을 경감시킬 수 있다.
- Progressive fusion of intermediate feature를 통해 네트워크는 풍부한 information으로 성능이 향상되며, adopted attention mechanism은 네트워크가 essential feature에 초점을 맞추도록 가이드한다. 더불어 AFB의 특징인 Residual in Residual(Residual Group에 Residual block이 있는것)를 통해 Network는 global structure과 high-frequency detail의 feature map을 추출할 수 있다.
-Shortcut과 global, local residual connection은 gradient vanishing문제를 해결하고 네트워크가 중요 feature를 학습하도록 돕는다.
댓글