FHDeNet: Full High Definition Demoireing Network (ECCV 2020)
-Authors: Bin He, Ce Wang, Boxin Shi, and Ling-Yu Duan
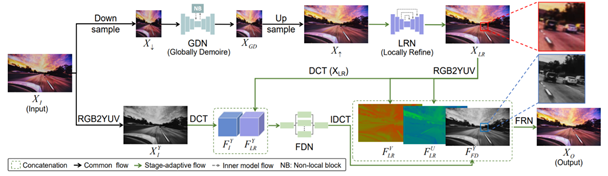
-본 논문에서는 현재까지 demoireing 기법들은 full high definition(FHD) image에서 실용적인 효과를 내지 못하며, 이를 해결하기 위해 global to local cascaded removal branch를 통해 다중 스케일로 모아레를 제거하고, frequency based high-resolution content separation branch를 통해 fine detail을 유지한다. 더불어 새로운 FHD moire image dataset을 생성했다.
이전 모아레 제거 기법들의 한계점
-이전 기법들은 receptive field가 100x100 size로 FHD moire image의 large-scale moire pattern을 잡아내는데 효과적이지 않다.
-Demoireing 작업은 high-resolution detail loss를 초래하는데, 이는 spatial domain에서 모아레 패턴과 edge의 difference를 무시하고 pixel modification을 하기 때문에 이미지가 over-smoothing된다.
-이전 기법들은 low-resolution morie dataset을 crop한 image로 학습되었기 때문에, high-resolution morie image에서 generalization 능력이 떨어진다.
-Global to local cascaded removal branch, receptive field를 넓히며 high-resolution에서 large-scale인 모아레 패턴을 해결한다. Global demoireing Network (GDN)과 Local Refinement Network(LRN) 2개의 네트워크가 연쇄적으로 이어지며 global에서 local level로 모아레 패턴을 각각 제거한다.
-Global demoireing Network (GDN), high-resolution input을 downsample한 후 dense block based auto-encoder(pooling operation 역할)에 입력한다. 이를 통해 기존 기법들의 receptive field가 100x100이었지만 GDN은 400x400으로 확장시킨다. 더불어 non-local block(strengthen long distance feature)을 추가하여 large-scale moire pattern의 전체 spatial connection을 강조한다. 즉 Downsampling을 통해 receptive field를 넓히고, non-local feature를 통해 large-scale moire를 global removal이 가능하게 된다.
-Local Refinement Network (LRN), GDN으로 global removal이 가능하지만, LRN을 통해 지역적 타켓팅도 필요하다. 따라서 stage-adaptive strategy(local enhancement)를 채택하여 regional refinement에 초점을 둔다. Stage-adaptive data flow는 figure 1에서 녹색 화살표로 표시되며, 학습 단계에서 local residue distribution에 집중한다. 또한 moire는 모아레가 포함된 지역의 밝기가 밝을수록 모아레의 강도가 더 강해진다. 따라서 mask-based algorithm을 통해 local residue의 학습 수렴속도를 가속화한다.
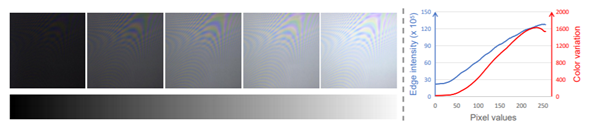
-Frequency based high-resolution content separation branch, moire를 제거하는 동안 fine detail을 유지하기 위해 frequency domain feature를 사용한다. Image를 downsampe하게 되면 image high detail요소가 사라진다. 따라서 모아레를 제거하며 적절히 fine detail을 유지하기 위해서는 input 영상에서 high-frequency pattern을 분리해야 한다. 먼저 high-resolution input image를 YUV color space에서 luminance (Y) channel을 추출한다. 이는 luminance가 각 pixel별 색의 강도를 측정하기 때문이다. 추출된 Y channel은 DCT transform을 통해 frequency domain으로 변환된다. DCT transform은 아래 Figure 3 참고. 또한 Figure 4를 통해 서로 다른 frequency band를 관찰할 수 있으며, 각 band별로 서로 다른 크기의 moire를 가지고 high-resolution detail을 포함한 것을 확인할 수 있다. 이렇게 서로 다른 frequency band를 모두 고려하기 위해 SE(Squeeze and Excitaiton) block을 FDN에 적용하여 각 채널 별로 서로 다르게 가지는 high-frequency moire pattern과 image detail을 구별할 수 있다. 이 output과 upper branch의 output을 DCT 변환을 하고 합치며 1X1 convolution을 통해 fusion된 결과물이 FDN으로 입력되고 결과물을 다시 IDCT를 통해 spatial domain으로 변환한다.
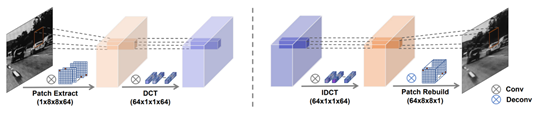
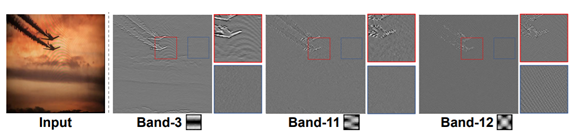
-Layer fusion and refinement, upper branch의 output은 모아레를 제거했지만 high-resolution detail이 없고, lower branch의 output은 luminance information을 보유했지만, chrominance information이 없다. 따라서 이 upper branch의 결과물을 YUV color space로 변환하여, U, V channel(contain chrominance)과 down branch의 output(luminance information)을 fusion하여 최종 결과 영상을 생성한다.
-Newly collected Full High Definition Morie image dataset, FHD camera phone으로 screen을 촬영한 새로운 Full High Definition Moire image dataset을 제안한다.


댓글